Forecasting methods for spatio-temporal data: robust evaluation and inference
A06 develops forecasting methods and forecast evaluation tools with a focus on panel data with a particular emphasis on robustness. It will develop forecasting methods and tools for testing forecast rationality and forecasting superiority, which take the effects of parameter estimation into account. The long-term goal are inferential comparison tools for general classes of prediction methods for spatio-temporal data.
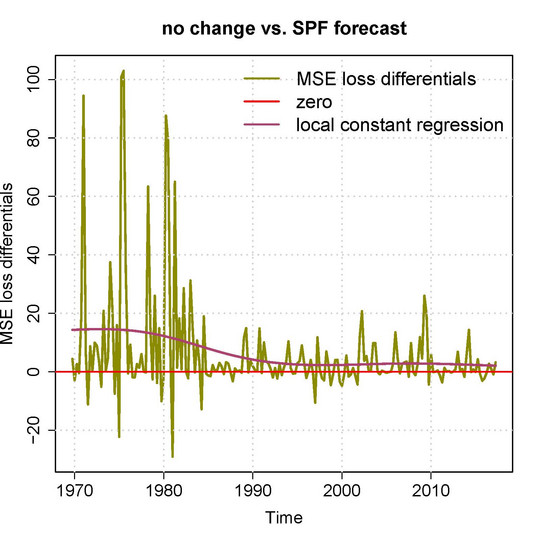
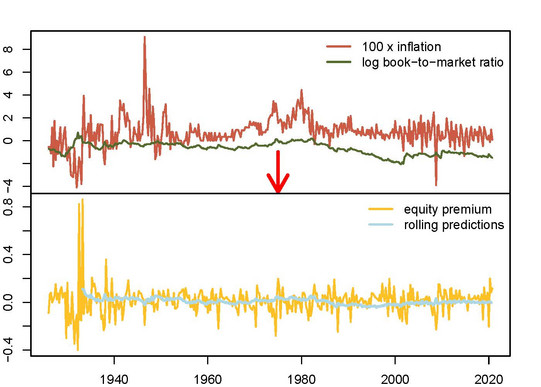
Project Leaders
Prof. Dr. Dr. Matei Demetrescu
Department of Statistics - Chair of Econometrics and Statistics
TU Dortmund University
Prof. Dr. Christoph Hanck
Faculty of Business and Economics - Chair of Econometrics
University of Duisburg-Essen
Summary
We develop forecasting tools able to cope with complex data structures that arise in panel data setups, in particular with any spatial relation between panel units. Moreover, for a given set of different, competing forecasting methods, we develop methodology that allows to test whether some method is superior to others as well as whether a given method makes rational use of available information.
We further consider functional time series models for cases where the data vary smoothly in a suitable spatial dimension. This includes both natural space for, e.g., energy production sites and "spaces" of forecasters relying on related and hence spatially dependent information sets. We explore the implications of such modeling for forecasting methods as well as assessment.
As robustness is required in virtually all leading applications, particular emphasis is placed on the development of procedures robust to nuisance features of the data. Along these lines, we provide forecasting methods that are robust to the effect of parameter estimation, as well as to data properties such as high, but unknown degrees of predictor persistence in time, dependence of unknown form across the spatial dimension, heteroskedasticity, coefficient heterogeneity across the panel as well as coefficient instability across time, and time-varying variances. To this end, we make use of suitable resampling, instrumental variables, local volatility estimation and shrinkage techniques.
An important aspect that will be addressed throughout this project is to explore such features for the improvement of efficiency of the methods relative to benchmark approaches.
Demetrescu, M., Hillmann, B. (2025). Gaussian inference in predictive regressions for stock returns. Journal of Financial Econometrics, 23(2), nbaf004. DOI: 10.1093/jjfinec/nbaf004.