Energy price shocks: identification, transmission, and induced technological change
C01 investigates strategies using structural vector autoregressive (SVAR)-type models for the identification and estimation of the impulse responses of energy price shocks from macroeconomic and sectoral time series. It analyzes whether higher energy prices speed up the development of energy-saving technologies. In the long run, the project develops methodologies for non-stationary and mixed-frequency data as well as novel identification strategies for SVARs with functional anticipated energy prices.
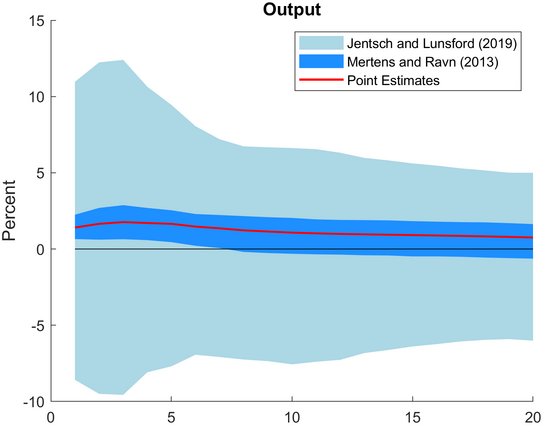
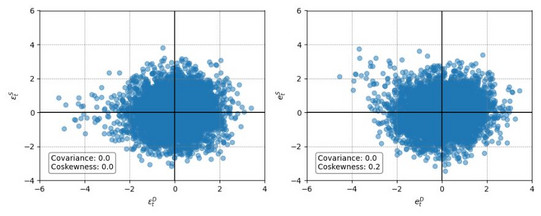
Project Leaders
Prof. Dr. Christoph Hanck
Faculty of Business and Economics - Chair of Econometrics
University of Duisburg-Essen
Prof. Dr. Carsten Jentsch
Department of Statistics - Chair of Business and Social Statistics
TU Dortmund University
Prof. Dr. Ludger Linnemann
Department of Business and Economics - Chair of Applied Economics
TU Dortmund University
Summary
The transition to a decarbonized economy will likely result in increased energy prices for maybe decades, until renewable energies will be available at a scale sufficient for a full transition. By taking spatial/regional information into account, we identify and estimate the macroeconomic effects of energy price shocks from aggregate multi-country and multi-sector time series data. This requires a solution to a fundamental identification problem: since energy prices also respond endogenously to variations in energy demand (e.g., due to the state of the business cycle), a causal analysis of the effects of energy prices requires the identification of truly exogenous supply side energy price shocks.
A large macroeconometric literature attempts to estimate the effects of oil price shocks, but often relies on debatable identification assumptions such as recursiveness. We develop new statistical identification procedures to causally estimate the effects of oil price shocks. Oil prices have the advantage that they are readily available as long time series at high frequencies, and they are highly correlated with the costs of other sources of energy. By leveraging the panel dimension of macroeconomic data, our goal is to make explicit use of spatial information via, e.g., country-specific shocks and transmission mechanisms to develop various new statistical identification procedures (based on, e.g., higher moments, volatility processes, or generative adversarial networks) to causally estimate the effects of oil price shocks, including accounting for, e.g., mixed frequencies. Further, we derive broader measures of energy costs and usage and will thus be able to identify and estimate the impact of energy cost shocks on aggregate output, employment and prices as well as the induced substitution between production factors and broader energy sources. We also produce novel estimates of the causal effects of energy price shocks on energy-saving technological progress, which is the key metric for the assessment of the overall macroeconomic cost of decarbonisation.
To assess estimation uncertainty associated with these identification strategies, we develop asymptotic distribution theory for the (parameter) estimates that enables the construction of valid confidence intervals for impulse response analysis. To improve the finite sample performance and to address the problem of estimating the usually large number of nuisance parameters in limiting distributions, we construct suitable resampling schemes making use of (residual-based) block bootstrap as well as multiplier bootstrap strategies. Suitably applied, these inferential procedures will be capable of handling typical data features such as temporal and spatial dependence in the panel, heteroskedasticity, mixed frequencies as well as non-stationarities. We also leverage the panel dimension typically available and make use of shrinkage approaches to reduce the typically large number of parameters in order to improve estimation accuracy.
Bruns, M., Keweloh S. A. (2025). Testing for strong exogeneity in proxy-VARs. Journal of Econometrics, 245(1-2). DOI: 10.1016/j.jeconom.2024.105876.
Hanck, C., Massing, T. (2024). Testing for nonlinear cointegration under heteroskedasticity. Econometric Reviews, 44(4), 512–543. DOI: 10.1080/07474938.2024.2429598.